The crawl, walk, run playbook for finance AI

- Crawl: Save time with repetitive tasks
- Walk: Automate simple financial analysis
- Run: Execute more complex analyses
- Get started with finance AI
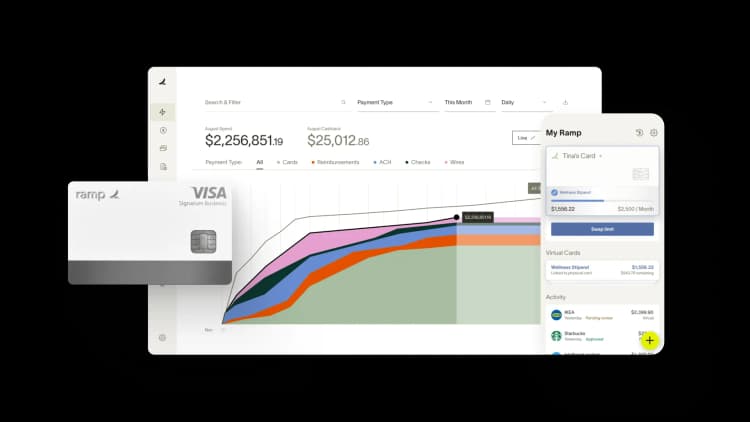
The most common concern I hear about finance AI is that people simply don’t know how to start using it.
We recently polled over 500 finance folks during a webinar and asked them to describe their comfort level with AI. Only 2% say they feel confident (aka “running”); 75% say they’re still learning the basics (aka “crawling”).
The good news is that it doesn’t take a computer science degree to start incorporating AI into daily finance work. Here is a “crawl, walk, run” framework for finance AI based on a recent workshop I hosted with Nicolas Boucher, founder of the AI Finance Club.
Crawl: Save time with repetitive tasks
You don’t need to build an AI model to get started with finance AI. In the crawl stage, Nicolas recommends that people start with the most accessible tool of all: ChatGPT.
Maybe you’ve tried ChatGPT and found its responses unhelpful. That’s likely due to unclear prompts. Instead of treating ChatGPT like Google, think of it as an assistant and use the “CSI framework”—Context, Specifics, and Instructions—to optimize your prompts.
Take a dunning letter, for example. If a human assistant were to write a dunning letter, they would need context. You have to give ChatGPT the same context. Rather than simply telling ChatGPT to write a dunning letter, write something like this: I am an accountant, and my client has an invoice that’s overdue by two months. Can you draft a communication to my client?
With the CSI framework, you’ll get output that is far closer to what you need. Even if you have to edit the dunning letter before sending it, ChatGPT will still save you time. It can also help with other simple tasks like researching accounting policies.
Walk: Automate simple financial analysis
In the walk phase, start using AI for financial analysis. Not only can ChatGPT help you create formulas, but it can also recommend analytical approaches based on your available data.
For example, let’s say you’re doing headcount analysis. You can upload a sample Excel file to ChatGPT and ask it to act as a McKinsey consultant and prepare a presentation for your board. You can even tell ChatGPT to include visualizations to support the analysis.
In this case, based on the column headings we provided, ChatGPT recommended that we analyze departmental headcount distribution, average salaries by department and position, and few others. It was able to produce in seconds what your team might have taken hours to do on their own.
Once you’re satisfied with ChatGPT’s recommendations for your sample data, you can prompt the tool to provide instructions on how to execute the same analysis in Excel. Apply these steps to your actual file to recreate the analysis and charts. Remember, you shouldn’t upload sensitive data to the standard version of ChatGPT.
Run: Execute more complex analyses
As wide-ranging as ChatGPT is, it has some limitations. Outside of data confidentiality, you can’t easily share your results (like the formulas that ChatGPT creates) with other people in your department for auditability. ChatGPT also can’t handle large datasets.
That’s where Python comes in. It is a common coding language that ChatGPT uses for its analyses. You can ask ChatGPT to show its Python code and run it in tools like Google Colab, Microsoft Azure, and Visual Studio to analyze as many private files as you need.
This approach solves the shareability problem. If you use Google Drive in your organization, Google Colab files can be shared the same way you would share a Google Doc or Sheet. Moreover, the Python code acts as an audit trail for your work and can be reviewed by others for accuracy. If you have questions about the code, you can even take a screenshot of the code in question and ask ChatGPT to explain it.
Get started with finance AI
Manual processes often bog down finance work, but it doesn’t have to be that way. Start reclaiming your team’s time and capacity with everyday AI tools like ChatGPT. Practice everyday and you will find new use cases.
Over time, explore other tools that automate expense management, auditing, variance analysis, and more. For example, Ramp’s AI can benchmark software pricing, screen expenses for anomalies, automate expense coding, and more.
In the workshop, Nicolas shares even more recommendations, including how you can tailor your AI approach based on your organization size. Check out the full session here.

“We’ve simplified our workflows while improving accuracy, and we are faster in closing with the help of automation. We could not have achieved this without the solutions Ramp brought to the table.”
Kaustubh Khandelwal
VP of Finance, Poshmark

“Our previous bill pay process probably took a good 10 hours per AP batch. Now it just takes a couple of minutes between getting an invoice entered, approved, and processed.”
Jason Hershey
VP of Finance and Accounting, Hospital Association of Oregon

“When looking for a procure-to-pay solution we wanted to make everyone’s life easier. We wanted a one-click type of solution, and that’s what we’ve achieved with Ramp.”
Mandy Mobley
Finance Invoice & Expense Coordinator, Crossings Community Church

“We no longer have to comb through expense records for the whole month — having everything in one spot has been really convenient. Ramp's made things more streamlined and easy for us to stay on top of. It's been a night and day difference.”
Fahem Islam
Accounting Associate, Snapdocs

“It's great to be able to park our operating cash in the Ramp Business Account where it earns an actual return and then also pay the bills from that account to maximize float.”
Mike Rizzo
Accounting Manager, MakeStickers

“The practice managers love Ramp, it allows them to keep some agency for paying practice expenses. They like that they can instantaneously attach receipts at the time of transaction, and that they can text back-and-forth with the automated system. We've gotten a lot of good feedback from users.”
Greg Finn
Director of FP&A, Align ENTA

“The reason I've been such a super fan of Ramp is the product velocity. Not only is it incredibly beneficial to the user, it’s also something that gives me confidence in your ability to continue to pull away from other products.”
Tyler Bliha
CEO, Abode
